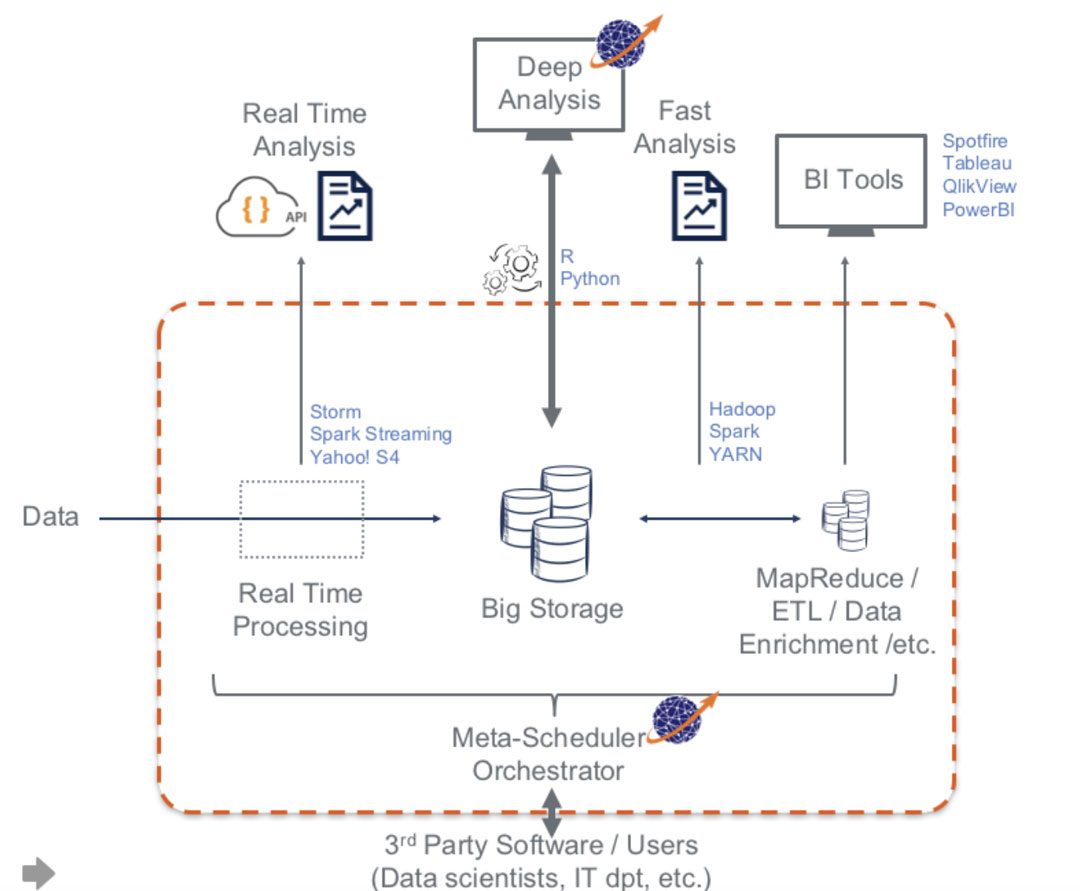
Whitepaper - 2018
Nowadays, we are awash in a flood of data. With a rapid growth of applications like social network analysis, semantic web analysis, bioinformation analysis, AI, IoT analysis, a large amount of data needs to be processed to improve accuracy and create a meaning. For example: Google deals with more than 24 PB of data every day (a thousand times the volume of all the publications contained by the US National Library); Facebook updates more than 10 million photos and 3 billion “likes” per day; YouTube accommodates up to 800 million visitors monthly (a video more than an hour uploaded every second); and Twitter is almost doubling its size every year, etc.
Consequently, Big Data processing represents diverse challenges at different time of the data journey. Some information needs to be extracted as soon as the data is created while other information may require a larger set of data to be meaningful. Even though many solutions have their own simple scheduler and resource manager, to organize all these activities, and optimize the parallelization of treatments, a comprehensive Orchestrator or Meta-Scheduler with Workflow capability is generally required.
We will get back to those orchestration aspects at the end of this White Paper, let start first with presenting the various kinds of Parallel Data processing used for Big Data.